Z-test explained
By Prachi Sinha
Reviewed by Vanessa Kintu
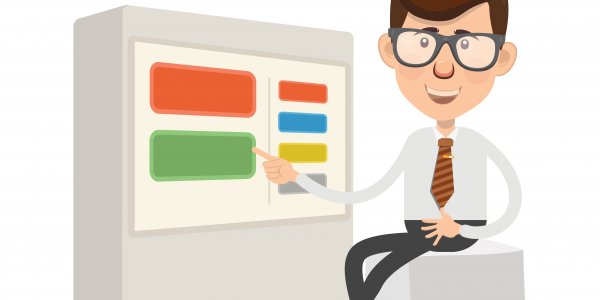
What is a Z-test? Typically it’s a test applicable on statistical data that follows a normal distribution. A Z-test is used for checking whether the mean of two known populations is equal to the other, provided the population variance is already known. Put simply, it carries out a hypothesis test in which the Z-test statistic follows a normally distributed data set.
There are certain factors critical to understanding the Z-test definition:
Sample size: The Z-test is conducted on a sample population size that is more than 30 individual data points. This is because it follows the Central Limit Theorem that states as the sample size becomes larger, the distribution of the sample will approximate a normal distribution, which is depicted as a bell curve – assuming all data sample points are identical in size,
Standard deviation: For an accurate Z-test, it’s mandatory that the population variance or standard deviation of the data set is already known.
Independent data points: The individual data points in the given data set should exist without any material relation to each other. A change in one data point shouldn’t affect the other.
Random selection: All independent data points within the sample size should have been selected at random from the population set.
Hypothesis setting: A Z-test means a statistical technique that’s adopted to test an alternative hypothesis against a null hypothesis within a given data set.
Steps in a Z-test process
There are typically five steps involved in the process of performing a Z-test.
Step 1: A null hypothesis and alternate hypothesis must be established. The former relates to the result researchers are looking to nullify or disapprove, while the latter is the hypothesis they’re trying to prove true for the given data set.
Step 2: An alpha or significance level must be chosen. In a typical Z-test example, an alpha level of 0.05 or 5% is chosen.
Step 3: A Z-table helps calculate what percentage of data is under a normal distribution curve at any given point. The next step is to figure out the Z-critical value in a Z-table.
Step 4: Calculate the Z-test statistic using the following Z-test formula:
Wherein: ‘ ̅x’ is the sample mean; ‘μ0’ is the population mean; ‘σ’ is the population standard deviation; and ‘n’ is the sample size or number of observations.
Step 5: By comparing the Z-test statistic against the Z critical value, you can decide whether the null hypothesis should be accepted or rejected.
Types of Z-tests
Ideally, there are two types of Z-tests: one sample Z-test and two sample Z-test. The former checks the difference between the population mean and sample mean; while the latter is used to find if a difference exists between the averages of two different sample data.
Difference between T-test and Z-Test
A T-test is also carried out to determine if there is a difference between the means of two sets of sample data. However, it differs from a Z-test in the following aspects:
In a T-test, the population variance is not given; whereas a Z-test would be conducted accurately only when the population variance is already provided for the sample data.
A T-test is only conducted for relatively smaller sample size data. To be specific, for a sample size exceeding 30 units, a Z-test is resorted to. For a T-test, the sample size should ideally contain five to 30 sample units only.
Related Terms
Latest video
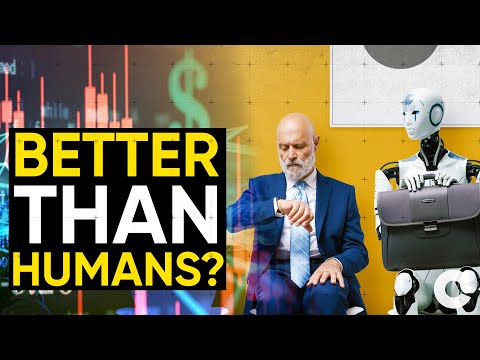